-
摘要: 遥感图像尤其是高分辨率(1~4 m)遥感图像在树种分类方面有着广阔的应用前景。利用主成分分析法对遥感数据去相关分析,然后通过对纹理提取过程的分析,探讨不同移动窗口大小对纹理特征的影响,以期为中山陵园风景区的森林调查提供依据,分类方法为经典的最大似然分类器。根据不同移动窗口大小的纹理因子相关性和对保持纹理信息丰富度的影响,来选择合适的窗口大小及纹理因子组合,以对树种分类精度的提高程度为评价标准。研究结果表明,利用窗口大小为19 19下的纹理信息可有效提高分类精度,总精度达到66%,Kappa系数达到0.59,比单纯的光谱信息最大似然法图像分类精度高,其中均值与均匀性、对比度、偏斜度纹理因子组合为最佳纹理组合,能有效减少数据冗余。高分辨率遥感数据纹理信息的运用为树种分类识别时的特征选择提供了有利技术参考。图4表3参19Abstract: Remote sensing images show a very promising perspective for distinguishing tree species,especially those with the very high resolution ranging from 1 to 4 m. However,the traditional methodology for classifying land cover types,solely depending on spectral features,while texture and other spatial information are neglected,has the weakness such as inadequately utilization of information,low accuracies of classification etc. Considering to the texture differences among forest species,it is more important for spatial information discription of high-resolution remote sensing image to improve the precision of textural features choosing. In this study,the factors to influence the nine textural features choosing were analyzed and the results showed that the moving window size was the main factor to affect the obtaining processes of textural features based on the gray level co-occurrence matrix (GLCM) method,and the imagery was then classified combining the maximum likelihood classification (MLC) method with the original spectral values and texture features. First,this study utilized a correlation analysis of the images from a principal component analysis. Second,through multiple information sources,including textual features derived from the data. For the high-resolution remote sensing image,the most proper moving window size was determined from 3 3 to 31 31. Classification of the major tree species throughout the study area (the SunYat-Sen Mausoleum in Nanjing) was undertaken using the MLC. Third,to aid forest research,classification accuracy was improved using the GLCM. According to correlations among textures and richness of the data,GLCM provided the best window size and textural parameters. Results indicated that the texture characteristics were add in the spectral characteristics to improve the precision of the results of the classification,19 19 window for best window. The total precision can reach 66.322 6%,Kappa coefficient is 0.5840. Each tree species has greatly improved accuracies of the classification. By the calculation of R2 values,the textural features of mean,homogeneity and correlation were chosen to be best combination for the size of 19 19 and the combination of skewness,homogeneity and mean was considered the most properly for the moving window size 19 19. Precision assessment of different textural combinations showed that VA,HO,CR combination with optimal moving window size (from 3 3 to 31 31) could evidently improve the classification precision for high-resolution remote sensing image. And the combination of mean,homogeneity,skewness,and contrast texture factors correlation can effectively reduce data redundancy,which obtained the similiar results. In the texture features,the mean is the most important factor and impacts the classification of the tree species. This method could solve problems of forestry type classification,tree species classification etc. It is much better than traditional method of based on pixel values. This procedure effectively reduced data redundancy and could assist in tree species classification.[Ch,4 fig. 3 tab. 19 ref.]
-
-
链接本文:
https://zlxb.zafu.edu.cn/article/doi/10.11833/j.issn.2095-0756.2012.02.010
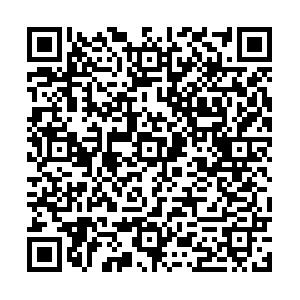
计量
- 文章访问数: 3401
- HTML全文浏览量: 200
- PDF下载量: 1548
- 被引次数: 0