Geographically weighted regression based on estimation of regional forest carbon storage
-
摘要: 森林碳储量与其调查因子之间的关系及其空间相关性特征是区域森林碳储量及其分布估计模型建立的基础,然而某一调查因子在不同空间位置对森林碳储量估计的影响程度存在差异。地理加权回归方法考虑了调查因子作用的空间异质性,进行局部回归估计。以浙江省台州市仙居县森林资源二类调查样地实测数据为数据源,利用地理加权回归方法结合陆地探测卫星系统Landsat TM影像数据进行森林碳储量及其密度的分布估计,并检验地理和海拔加权回归在地势平缓的区域是否可用。结果表明:①仙居县森林地上部分总碳储量3.132106 Mg,与样地实测统计得到的全县碳储量3.192106 Mg相差1.880%;地理加权回归模型估计结果与实际碳密度分布情况相一致,研究区内碳密度的取值范围为0~89.964 Mghm-2,保留了70%以上的空间异质性特征;基于地理加权回归的森林地上部分碳储量估计方法是有效的,地理加权回归在区域碳储量方面估计结果合理且精度较高。②在地势较为平缓的地区,海拔对植被的影响不显著,地理和海拔加权回归并不适用;若将海拔作为解释变量加入建模,能够提高估计精度,但存在多重共线性问题。图6表9参26Abstract: Global climate issues have confirmed the irreplaceable role forest carbon stocks play in the global carbon cycle. To research whether the geographically weighted regression (GWR) model method which considers the role of survey factors spatial heterogeneity and establish the local regression model, can improve the estimation accuracy of forest carbon stocks, instead of the more commonly used methods of global regression model such as ordinary least squares analysis(OLS), we used forest management inventory data in Xianju County, Zhejiang Province, combined with Landsat TM image data developing local models using GWR to estimate forest carbon stock and its density. Available of geographically and altitudinal weighted regression (GAWR) model was then tested in smooth terrain. Analysis is included comparison to traditional regression and co-kriging interpolation. Results showed that the total forest aboveground carbon stocks estimated by the GWR(T) model for Xianju County were 3.132 106 Mg, and carbon density ranged from 0 to 89.964 Mghm-2 with a mean value of 15.555 Mghm-2. Meanwhile, the total forest aboveground carbon stocks calculated from diameter measurements were 3.192 106 Mg with a mean value of 15.854 Mghm-2. The overall result from GWR(T) model was lower than diameter measured by 1.880%, R2 = 0.654 (P<0.01), and carbon density distribution was consistent with the actual situation. The estimated results also had a higher accuracy with the RRMSE = 9.802 (P<0.01) than traditional regression method with the RRMSE = 15.033 (P<0.01) and co-kriging interpolation method with the RRMSE = 16.427 (P<0.01). GWR method can effectively estimate the regional forest aboveground carbon stocks reasonably and accurately, however, the GAWR model is not applicable for the areas with smooth terrain. Adding altitude as an explanatory variable in the modeling could improve estimation accuracy but would in turn create a multi-collinearity problem.[Ch, 6 fig. 9 tab. 26 ref.]
-
-
链接本文:
https://zlxb.zafu.edu.cn/article/doi/10.11833/j.issn.2095-0756.2015.04.002
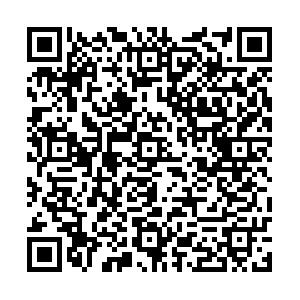
计量
- 文章访问数: 2507
- HTML全文浏览量: 316
- PDF下载量: 680
- 被引次数: 0