Chestnut browning detected with near-infrared spectroscopy and a random-frog algorithm
-
摘要: 为了实现板栗Castanea mollissima的快速自动分选,研究了基于近红外光谱技术的褐变板栗无损检测方法。首先采用Antaris Ⅱ傅里叶变换近红外光谱仪获取70个正常板栗和110个褐变板栗的近红外光谱(1 000.00~2 500.00 nm),比较了不同光谱预处理方法对褐变板栗识别的影响,随后采用一种新的变量选择方法即随机青蛙算法(random frog)提取与板栗褐变相关的特征波长变量,最后基于特征波长建立和比较了褐变板栗识别的偏最小二乘?鄄线性判别分析模型(PLS-LDA)和最小二乘-支持向量机(LS-SVM)模型。结果显示:经标准正态变量变换(SNV)预处理和随机青蛙算法优选的23个特征波长所建LS-SVM模型的性能最优,该模型对测试集的敏感性、特异性和识别正确率分别为0.92,1.00和95.00%。随机青蛙算法可以有效筛选重要的特征变量,不仅能简化模型,而且可以提高识别准确率和识别速度。图4表3参22
-
关键词:
- 经济林学 /
- 近红外光谱 /
- 褐变 /
- 随机青蛙算法 /
- 最小二乘-支持向量机 /
- 偏最小二乘?鄄线性判别分析 /
- 板栗
Abstract: To develop a calibration model for rapid, accurate and nondestructive detection of chestnut browning with peeled chestnut Castanea mollissima by using near infrared spectroscopy technology. Seventy normal chestnuts and 110 browning chestnuts were prepared, and their diffuse reflectance spectrums were collected in the wavelength range from 1 000.00 to 2 500.00 nm. Spectral pretreatment methods, including standard normal variate (SNV), multiplication scattering correction (MSC), first derivative (FD), second derivative (SD), and detrend, were used and compared first. Then random-frog algorithm was applied to select effective wavelengths (EWs) from the SNV pretreated spectrum. Afterward, a partial least squares-linear discriminant analysis (PLS-LDA) and a least squares-support vector machine (LS-SVM) model were established to classify the browning chestnuts based on EWs, and the results were compared based on sensitivity, specificity and accuracy. For the validation set, the sensitivity, specificity and accuracy obtained by EWs-LS-SVM were 0.92, 1.00 and 95.00%, respectively. The results were better than those of full-PLS-LDA model,full-LS-SVM model and EWs-PLS-LDA model. Also, the random-frog algorithm effectively selected important wavelengths and simplified the discrimination model improving precision and recognition speed. The overall results demonstrate that random-frog algorithm is a powerful tool to select the efficient variables, and EWs-LS-SVM is excellent for the spectral calibration. [Ch, 4 fig. 3 tab. 22 ref.] -
-
链接本文:
https://zlxb.zafu.edu.cn/article/doi/10.11833/j.issn.2095-0756.2016.02.019
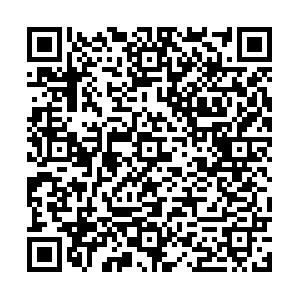
计量
- 文章访问数: 3093
- HTML全文浏览量: 341
- PDF下载量: 580
- 被引次数: 0